Predictive maintenance is a revolutionary concept in the manufacturing industry. Not only has it proven integral to substantially extending the lifespan of CNC machines and critical manufacturing equipment, but it also promotes productivity, efficiency, and uptime. It achieves this by relying on predictive models, which use previously collected and real-time sensor data to predict potential failures. Predictive maintenance departs from traditional methods.
Previously, manufacturing plants would rely on preventive and relative maintenance, which, though beneficial, disadvantageously caused – and still cause – productivity losses due to costly, unexpected breakdowns and downtime. However, as technology evolved and enabled the integration of advanced methods, a shift from the traditional model began to address these issues. As more and more workshops and plants embrace predictive maintenance, the resulting benefits are becoming clear for all. To put it simply, predictive maintenance has proven to be a significant game changer for the longevity of CNC machines and manufacturing equipment. This article details exactly how this has been achieved.
Table of Contents
Understanding Predictive Maintenance
What is Predictive Maintenance?
Predictive maintenance is a type of maintenance that involves tracking a CNC machine’s performance, status, and health as the machining or fabrication process is ongoing. With predictive maintenance, manufacturing plants use sensors and other advanced technologies to monitor and test different parameters and conditions such as temperature, vibration characteristics, bearing speed, lubrication, and sound.
These tools detect deviations from normal operations, sending real-time alerts if the parameters do not fall within the specified range. This way, the tools and technology alert the operator to a potential future breakdown. In actuality, predictive maintenance adopts a predictive model, which uses probability to estimate the time potential failures may occur.
To better discuss predictive maintenance, we will examine how it compares to other maintenance approaches and strategies.
Maintenance Approaches and Strategies
Preventive Maintenance
Preventive maintenance entails proactively performing fixed-in-time or scheduled routine maintenance operations or inspections to fix the issues before they occur. This maintenance approach helps prevent breakdowns or failures. It follows a schedule that is based on prior failure experience or data collected by the machine’s manufacturer.
This means that the only factor that technicians consider before undertaking any maintenance task is the time that has elapsed since the previous maintenance operation. Nonetheless, preventive maintenance helps technicians identify in advance the frequent causes of failures and breakdowns, either from experiments or experience.
Reactive or Corrective Maintenance
Also known as corrective maintenance, reactive maintenance involves servicing a CNC machine only when it breaks down, fails, or stops working. This maintenance approach is intended to maximize the productive manufacturing time. However, this advantage can be short-lived as this manufacturing approach also introduces issues such as unexpected irreversible damage due to the complete failure of the machine’s components.
As a result, it can cause unknown amounts of downtime, especially if the failure of one component damages more components. However, the repair work and the loss of productive manufacturing time can prove expensive in the long run. It can even harm customer relationships due to missed deliveries.
Predictive Maintenance
Unlike other maintenance approaches, predictive maintenance has a real-time attribute to it. It relies on data generated by the sensors in real time. It then uses predictive models to identify patterns in the data that indicate a potential anomaly. If the patterns point to an impending breakdown, the system posts a notification or alert. Technicians then take over by scheduling the much-needed service. Typically, predictive maintenance is used alongside other maintenance approaches to minimize maintenance costs and eliminate unplanned downtime.
Techniques and Technologies in Predictive Maintenance
Predictive Maintenance Techniques
A comprehensive predictive maintenance program includes multiple monitoring and diagnostic techniques. These techniques are deployed based on the type of machinery being monitored or operated. The predictive maintenance techniques include:
- Vibration monitoring
- Thermography
- Tribology
- Visual inspection
- Ultrasonics
- Nondestructive testing techniques
Vibration Monitoring and Analysis
As the name suggests, vibration analysis monitors the levels of vibrations that a machine produces to detect anomalies. It is a common technique that detects loose, misaligned, and imbalanced parts in rotary CNC machines. In this type of analysis, instruments monitor vibrations whose frequencies range between 1Hz and 30,000Hz.
Thermography
Thermography is a predictive maintenance technique that entails monitoring the surface temperature of CNC machines, such as 3D printers and other machinery, to determine operating conditions. This technique uses instrumentation that monitors how much infrared energy a machine emits. By design, machines operate optimally within a given range of temperatures. Anything higher or lower than the set range is considered a thermal anomaly. Thus, by detecting such anomalies, predictive maintenance instrumentation alerts technicians. The technicians then work to locate and define the cause of the problem, nipping it in the bud before it can cause failure.
Tribology
Tribology is the study of friction, wear, and lubrication. Against this backdrop, tribology as a predictive maintenance technique entails tracking surfaces within the CNC machine that, due to their relative motion, cause friction and, therefore, require lubrication. Two approaches are often used in tribology: lubricating oil analysis and wear particle analysis.
Lubricating oil analysis is used to determine the condition of the oils used in the equipment by taking oil samples. However, unlike other predictive maintenance techniques, which can be used to identify failure modes or the actual cause of problems in machines, lubricating oil analysis cannot. Instead, it is mainly used to conserve and extend the useful life of lubricating oils. It also helps determine the best and most cost-effective interval to undertake oil changes and as a quality control exercise.
On the other hand, wear particle analysis focuses on the particles found in the sample of lubricating oil. It specifically analyzes their quantity, size, composition, and shape with the aim of pointing out the wearing condition of the machine’s parts.
Visual Inspection
In visual inspection, technicians perform daily checks of CNC manufacturing systems. This predictive maintenance technique aims to identify potential failures or problems associated with maintenance that could adversely affect production costs, the quality of the parts, and the machine’s uptime or reliability. Even with the advent of Industry 4.0, visual inspections are still applicable and should be included even in modern predictive maintenance programs.
Ultrasonics
Also known as sonic acoustical analysis, ultrasonics is a category of noise analysis that monitors noise frequencies beyond 30,000Hz. These frequencies are often converted into visual signals, making the trend easy to visualize. In fact, because of the signals, technicians can document the ambient noise levels in the workshop or manufacturing plant. Using this as the baseline, they can easily detect anomalies such as noise level spikes.
These anomalies can result from under-lubricated or worn-out bearings. Additionally, the ability to monitor frequencies above 30,000Hz makes ultrasonics ideal for such applications as detecting leaks. Usually, leaking fluids cause high-frequency noise due to compression or expansion as they flow through the crack or orifice. Unfortunately, you cannot use ultrasonics to diagnose or establish the cause of the identified problem.
Nondestructive Testing Techniques
Nondestructive testing techniques, such as electrical testing, can be used to identify problems in CNC machines. They are used to determine the integrity of insulation, electrical impedance, and electrical resistance. However, these techniques are often too costly to justify their complementary use in predictive maintenance programs. Plus, they cannot be applied in a broad range of applications.
Technologies Used in Predictive Maintenance
The advent of Industry 4.0, which is centered around digitizing the manufacturing sector, including how plants and workshops carry out maintenance and servicing, expanded what predictive maintenance programs encompass. The technologies used in predictive maintenance now include:
Sensors
Sensors collect data on physical parameters such as rotation speed, temperature, vibrations, flow, fluid characteristics such as compression, expansion, and more. There are two types of sensors applied in predictive maintenance;
- Process sensors: Machine manufacturers integrate them into their machines to monitor various properties during operations.
- Test sensors: They are added by manufacturing plants or workshop owners specifically to detect anomalies. For instance, you can add accelerometers to identify irregular vibrations.
Scanners
In some cases, scanners can be used to monitor certain parameters. For instance, infrared instruments known as line scanners measure temperature. They provide a one-dimensional scan of the comparative heat radiated by the machine. However, scanners are not widely used in predictive maintenance of CNC machines.
Machine Learning and Artificial Intelligence
The role of artificial intelligence (AI) in design, manufacturing, and other industries is expanding. AI broadly encompasses creating computer systems that simulate human intelligence. It is quite a broad field comprising, among others, components such as machine learning (ML). ML focuses on creating programs known as models that learn patterns in data and use the learnings to identify patterns within new data. Backed by the ability to identify patterns, the models make intelligent decisions without additional and obvious programming.
In the context of predictive maintenance, where sensors collect data about various parameters of the CNC machines, AL and ML play a crucial role. Specifically, the models, known as predictive models, created with the help of AI and ML, can identify patterns in new sensor data, facilitating the prediction of potential failures.
Industrial Internet of Things (IIoT)
The Internet of Things (IoT) broadly extends computing capabilities and network connectivity to components or objects that may otherwise not be regarded as computers. By connecting the various objects and components, IoT allows for data mining and access to the data collected. It also facilitates the remote management of data. IoT can be applied in a myriad of industries.
IoT is called the Industrial Internet of Things (IIoT) when it is incorporated in manufacturing plants and workshops. It brings together a number of elements and domains, including traditional automation and machine-to-machine communication, big data and machine learning, software, and systems that integrate computational and physical components to control and monitor physical processes. By incorporating these domains, IIoT facilitates data collection from CNC machines, as well as its subsequent analysis and use, often in real time, to detect incipient problems and improve operations.
Cloud and Edge Computing
Cloud computing provides centralized, off-site storage and advanced processing capacity. It is advantageous in many ways. For instance, it lowers the cost associated with operating and maintaining powerful computers/servers that handle storage and processing.
Moreover, cloud computing supports virtualization and parallel processing. It also offers unmatched data security, especially if you choose reputable service providers. Combined, these advantages facilitate efficient processing of large volumes of data. By using cloud solutions, you can rest assured that there is enough bandwidth and computing power to train complex predictive models on a variety of conditions.
Cloud computing is nevertheless not always perfect. In fact, it is limited by speed and latency issues. It takes time to send large volumes of data hundreds or thousands of kilometers away to data centers and more or less equal time to receive responses once the data has been processed. Latency may not be an issue for a majority of cloud computing applications. However, in predictive maintenance where real-time responses are essential, latency can be a source of unreliability.
To deal with this limitation, edge computing is used. It reduces the amount of data transmitted to the cloud by processing it closer to where it is generated. This means the data does not have to travel long distances to data centers to be processed.
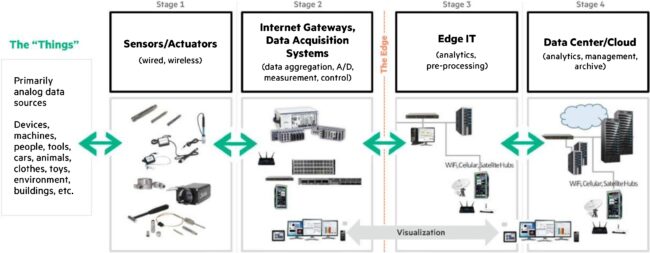
IIoT Solutions Architecture (source)
Implementing Predictive Maintenance in CNC Machines
If you are looking to integrate predictive maintenance in your CNC machine workshop, it pays to take a strategic approach. To enjoy the accompanying benefits, you must consider various factors and elements. To help you in the journey and to ensure you have checked all the requisite boxes, here are the five steps you should follow whenever you want to implement predictive maintenance:
- Create a predictive maintenance plan or program
- Get input from management and technicians
- Choose a partner or partners
- Build a predictive model
- Install the predictive maintenance technologies and deploy the model
We have discussed each of these steps in greater detail below.
Create a Predictive Maintenance Plan or Program
CNC machines vary from one to another. Based on the technology employed, some feature more moving parts than others. Some generate more heat than others, while for some, vibrations are the rule rather than the exception. Given these differences, predictive maintenance techniques and approaches will vary. This, in turn, will translate to differing predictive maintenance programs.
Based on the foregoing, creating tailor-made plans for each manufacturing plant and CNC machine is important. Such plans will, of course, capture the exact conditions of the machine rather than taking a one-size-fits-all approach. Because you understand your workshop’s situation, you also recognize which machine conditions, if disrupted, would be extremely costly for your business. Working together with teams drawn from multiple departments, you can draw up a plan to access the data that will show, in real time, the very operation-critical machine conditions.
Get Input from Management and Technicians
However, a plan is useless without widespread acceptance – including from management – or without feedback from its intended user. For this reason, the first step would entail getting the green light from management, if the idea did not originate from the top of the organizational hierarchy. One of the approaches you could use is listing the goals and benefits of the predictive maintenance program.
Secondly, it is equally important to ask your technicians for comments on whether or not they think the plan will work and how to improve aspects they deem unviable. After all, a successful predictive maintenance program depends on the people who will be using the technologies. Using their feedback, you can modify aspects of the program, optimizing them to align with the end-users’ preferences. Getting the technicians to buy into the predictive maintenance plan will ramp up their confidence levels in the data generated by the technologies.
Choose a Partner
Predictive maintenance in CNC machining relies on data. And in a typical plant where CNC machines run around the clock and are equipped with a large number of sensors for data collection, the storage and component management requirements are equally high. It would be illogical for such a company to manage all these tasks in-house, especially when they lack the specialized expertise. For this reason, it is crucial to choose a partner or multiple partners, each specializing in a specific aspect of the predictive maintenance ecosystem.
For instance, you might engage a cloud storage company for data architecture, design, governance, storage, and management; enlist model-building experts to craft predictive models; hire simulation experts, and so forth. You could also look for providers of quality sensors and actuators, not to mention management software. And given that you are starting out, you may not have the accurate data on which to train the predictive model, yet a third-party provider or the original equipment manufacturer may have it. Therefore, you could engage companies that will provide access to accurate training data.
Build a Predictive Model
After identifying the right partners, collaborate with them to develop a predictive model. The model will use the data collected from sensors and institutional knowledge of your CNC machines’ or machine’s conditions to predict future breakdowns. Such models will typically look at trends in the data, flagging any abnormal change or outliers as an indicator of incipient failure. Of course, not all changes are indicative of impending breakdowns. Some may point to factors that, if dealt with, can improve product quality.
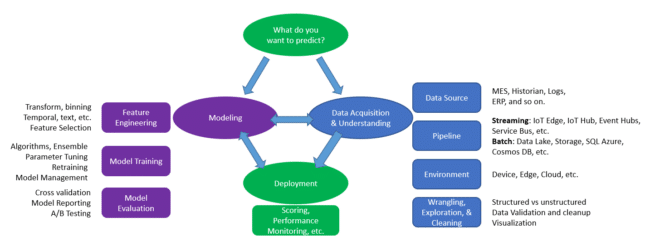
Predictive Model Delivery Methodology (source)
Install Predictive Maintenance Technologies and Deploy Models
The final step in implementing predictive maintenance is installing and deploying the technologies and predictive models. By deploying these critical components, you initiate the program and start reaping the benefits of integrated predictive maintenance systems in CNC machines.
Benefits of Predictive Maintenance in CNC Machines
Implementing predictive maintenance in CNC machining carries the following advantages:
1. Reduced Cases of Breakdowns
Predictive maintenance anticipates failures. It, therefore, enables technicians to diagnose and repair the cause before the problem escalates to a full-blown and unexpected breakdown.
2. Decreased Downtime
Unexpected breakdowns lead to unplanned downtime, which can negatively impact customer relationships. Such downtimes reduce productivity and, sometimes, even the product quality. Moreover, if the nature of the failure is extensive, the downtime can extend for an unknown period. Fortunately, predictive maintenance prevents these issues by anticipating potential failures. It allows technicians to schedule maintenance works, dealing with the problem early on. According to PTC, this maintenance approach decreases unplanned downtime by up to 30%.
3. Better Planning
A reliable predictive model offers accurate predictions. This accuracy manifests in the short interval between the estimated failure and the actual functional breakdown. In this regard, such a model improves planning. It informs technicians and service teams precisely when to plan maintenance.
4. Improved Productivity
Predictive maintenance of CNC machines maximizes uptime and guarantees peace of mind. Teams can operate without the fear of unforeseen breakdowns, allowing them to accept as many orders as the machinery can handle.
5. Increased Service Resolution
The predictive model lets them know the exact cause of the problem. As a result, it reduces the time taken to resolve the issue. As a result, according to PTC, a reliable model reduces the time on site by up to 75%. Moreover, with predictive maintenance programs backing the work of technicians, they resolve service requests faster by up to 83%.
6. Enhanced Safety
Unexpected failures can create hazardous workspaces. But by accurately forecasting when a failure is expected to occur, the models allow you to evacuate the area surrounding the machine when it breaks down. However, it does not always have to come to this point. Technicians can service the equipment before the expected failure or the machine becomes a workplace hazard.
Challenges and Solutions in Predictive Maintenance
1. Cost
Predictive maintenance technologies such as sensors can be costly. Fortunately, the cost of electronics has been dropping while their inherent capabilities have been increasing. This trade-off is increasingly making predictive maintenance of CNC machines more cost-effective.
2. Inadequate Training Data
A predictive model should be capable of accurately detecting potential failures. However, the model is only as accurate as the training data used. This means that the data should be all-encompassing. It should detail all aspects of the machine’s behavior and conditions, from how it functions normally, which is known as its signature, to how it behaves when there is an impending problem or outright breakdown. If the training data does not capture all aspects, then the model cannot forecast future failures correctly.
Unfortunately, this comprehensive data is rarely available because many companies do not maintain detailed records of past failures. Moreover, these manufacturers do not always supply or use process sensors to monitor certain events. These shortcomings make the training of the models quite challenging.
To solve this challenge, companies must maintain records of failures. Furthermore, they should use all the sensors incorporated into their machines to monitor all phenomena envisioned by the manufacturers.
3. Unreliable Predictive Models
Before the predictive model is refined to boost its reliability and accuracy, the time interval between the estimated failure and actual functional breakdown may be quite huge. This is untenable from a cost perspective; it can force teams to more frequently schedule unnecessary maintenance operations. It goes without saying that unnecessary maintenance operations can considerably impact productivity.
To solve this issue, it is necessary to minimize this interval. This can be achieved by collecting as much training data as possible. This data should include accurate depictions and descriptions of the machine’s conditions at different levels of performance and health.
4. Data Fragmentation
Tracking the right parameters can sometimes involve using multiple sensors. And while the ideal setup would have the sensors working in concert, this is not always the case. The sensors often tend to work in isolation, generating fragmented data. This often means that the data is then sent to different systems, known as data islands, and is stored using a variety of formats. While the data is theoretically usable, it cannot be easily and practically integrated as real-time training data, for example. As a result, the fragmented data cannot enrich the existing sensor data towards enhancing the accuracy of predictions.
Leveraging cloud and edge computing solutions mitigates these issues. You can set up all the sensors to send data to centralized repositories, eliminating unconnected data islands. This way, the sensors are architected to work together even when they are not directly interconnected.
Conclusion
Predictive maintenance of CNC machines and other manufacturing equipment has been a game-changer. It has been instrumental in extending the lifespan of machinery. Compared to conventional maintenance approaches like preventive and reactive maintenance, predictive maintenance has been shown to decrease unplanned downtime by 30% and the time on site by up to 75%. Its use also leads to faster resolution of breakdowns by as much as 83%, thus boosting worker productivity. The benefits of predictive maintenance of CNC machines are undeniable. But its implementation is often affected by data fragmentation, unreliable predictive models, cost, and inadequate training data. Fortunately, you can deal with these problems.
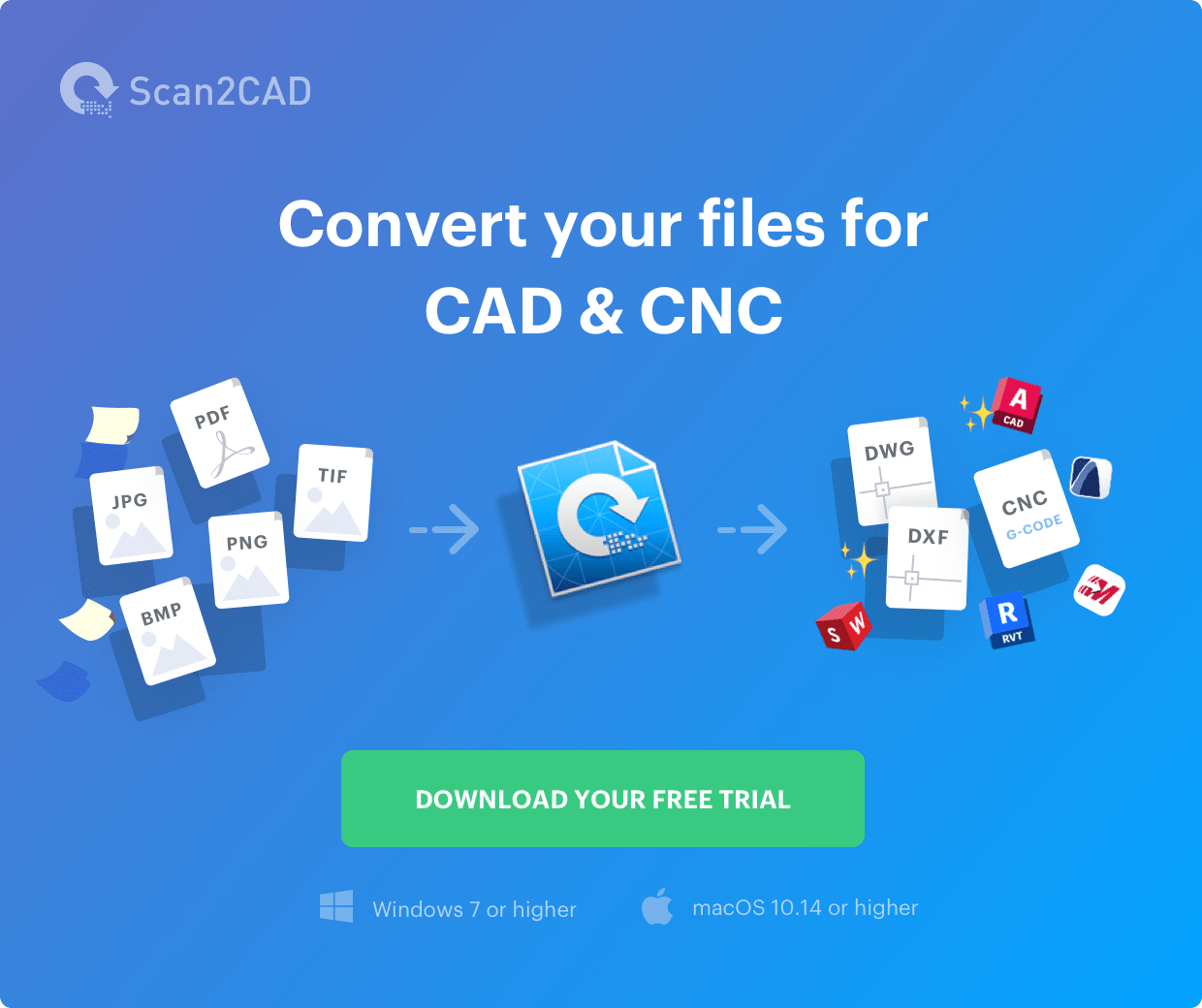